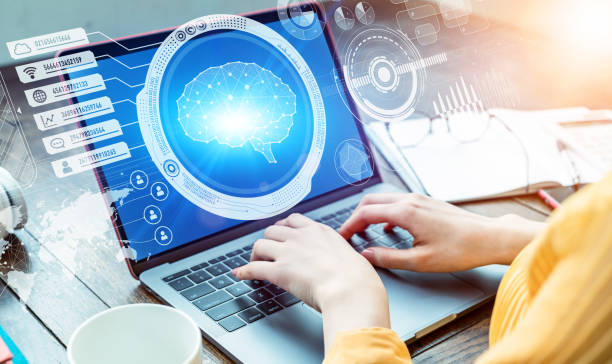
Artificial Intelligence (AI) has emerged as a transformative force, reshaping industries and redefining the way we interact with machines. One of the fascinating outcomes of this AI revolution is the surge in the adoption of edge computing. This paradigm shift is revolutionizing the way data is processed, analyzed, and utilized, bringing about a new era of efficiency, speed, and connectivity.
Understanding Edge Computing:
Edge computing represents a decentralized approach to data processing, where computation is performed closer to the source of data generation rather than relying solely on centralized cloud servers. This proximity to the data source reduces latency, enhances real-time processing capabilities, and minimizes the need for extensive data transfer to and from remote data centers.
The Role of AI in Sparking Edge Computing Adoption:
- Real-time Decision Making:
AI applications often require rapid decision-making based on real-time data analysis. Edge computing enables these AI algorithms to operate locally, ensuring quick responses without the delays associated with cloud-based processing. This is particularly crucial in applications like autonomous vehicles, industrial automation, and healthcare, where split-second decisions can make a significant impact. - Bandwidth Efficiency:
AI algorithms, especially those involving video analytics, generate massive amounts of data. Transmitting all this data to centralized cloud servers can strain network bandwidth and incur considerable costs. Edge computing allows AI models to process data locally, reducing the need for extensive data transfers and optimizing bandwidth usage. - Enhanced Privacy and Security:
Edge computing addresses concerns related to data privacy and security by keeping sensitive information closer to its source. AI models can process data on the edge device itself, minimizing the risk of data breaches during transit to remote servers. This is crucial in sectors such as healthcare and finance, where data confidentiality is of paramount importance. - Improved Latency:
In applications where low latency is critical, such as augmented reality (AR) and virtual reality (VR), edge computing ensures that AI algorithms can operate with minimal delay. This low-latency environment is essential for delivering seamless and immersive user experiences. - IoT Integration:
The Internet of Things (IoT) ecosystem, characterized by interconnected devices, sensors, and actuators, generates vast amounts of data. AI-powered edge computing facilitates the processing of this data at the edge, enabling more efficient and responsive IoT applications. This convergence of AI and IoT is driving innovation across various industries.
Case Studies:
- Autonomous Vehicles:
AI algorithms in autonomous vehicles rely on real-time data processing to make split-second decisions. Edge computing allows these vehicles to process sensor data locally, ensuring quick responses to changing road conditions and traffic scenarios. - Healthcare Monitoring:
Wearable devices equipped with AI algorithms for health monitoring can utilize edge computing to analyze data locally. This ensures timely detection of anomalies and reduces the dependency on continuous data transmission to central servers.
AI at the Edge: Gateway to Innovation
The symbiotic relationship between AI and edge computing is shaping the future of technology. As AI applications continue to proliferate across diverse industries, the demand for efficient, low-latency processing is driving the widespread adoption of edge computing. This dynamic duo is not just enhancing the capabilities of existing technologies; it is laying the groundwork for unprecedented innovations that will reshape the way we live, work, and interact with the digital world. The synergy between AI and edge computing is a testament to the transformative power of technology, propelling us into an era where intelligence and computation converge at the edge of possibility.